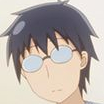
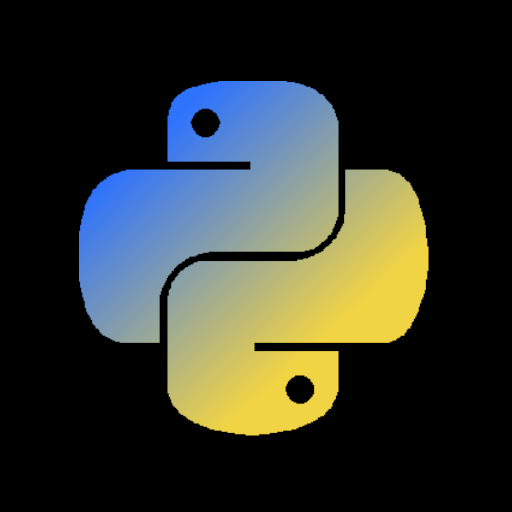
I’m most excited about the new REPL. I’m going to push for 3.13 upgrade as soon as we can (hipefully early next year). I’ve messed around with rc1 and the REPL is great.
Do you know why pytest was taking up so much RAM? We are also on 3.11 and I’m probably going to wait until 3.13 is useable for us.
Thanks for sharing. We use all pytest-style tests using pytest fixtures. I’ll keep my eyes open for memory issues when we test upgrading to python 3.12+.
Very helpful info!